Working Paper - Implications of Data Anonymization on the Statistical Evidence of Disparity
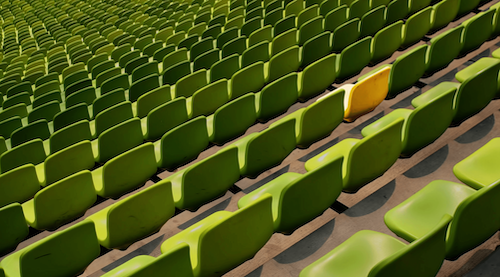
We posted on SSRN a working paper by Heng Xu and Nan Zhang, addressing the implications of data anonymization on the statistical evidence of disparity.
Abstract: Research and practical development of data anonymization techniques has proliferated in recent years. Yet limited attention has been paid to examine the potentially disparate impact of privacy protection on underprivileged sub-populations. This study is one of the first attempts to examine the extent to which data anonymization could mask the gross statistical disparities between sub-populations in the data. We first describe two common mechanisms of data anonymization and two prevalent types of statistical evidence for disparity. Then, we develop conceptual foundation and mathematical formalism demonstrating that the two data anonymization mechanisms have distinctive impacts on the identifiability of disparity, which also varies based on its statistical operationalization. After validating our findings with empirical evidence, we discuss the business and policy implications, highlighting the need for firms and policy makers to balance between the protection of privacy and the recognition/rectification of disparate impact.
Here is the link to the working paper. We are grateful for the helpful feedback and comments from the participants of the Harvard Privacy Tools Project Working Group Seminar and the 2020 Privacy Law Scholars Conference, where the paper was previously presented.