Talk at UMD: Privacy Contextualization: the Good, the Bad, and the Ugly
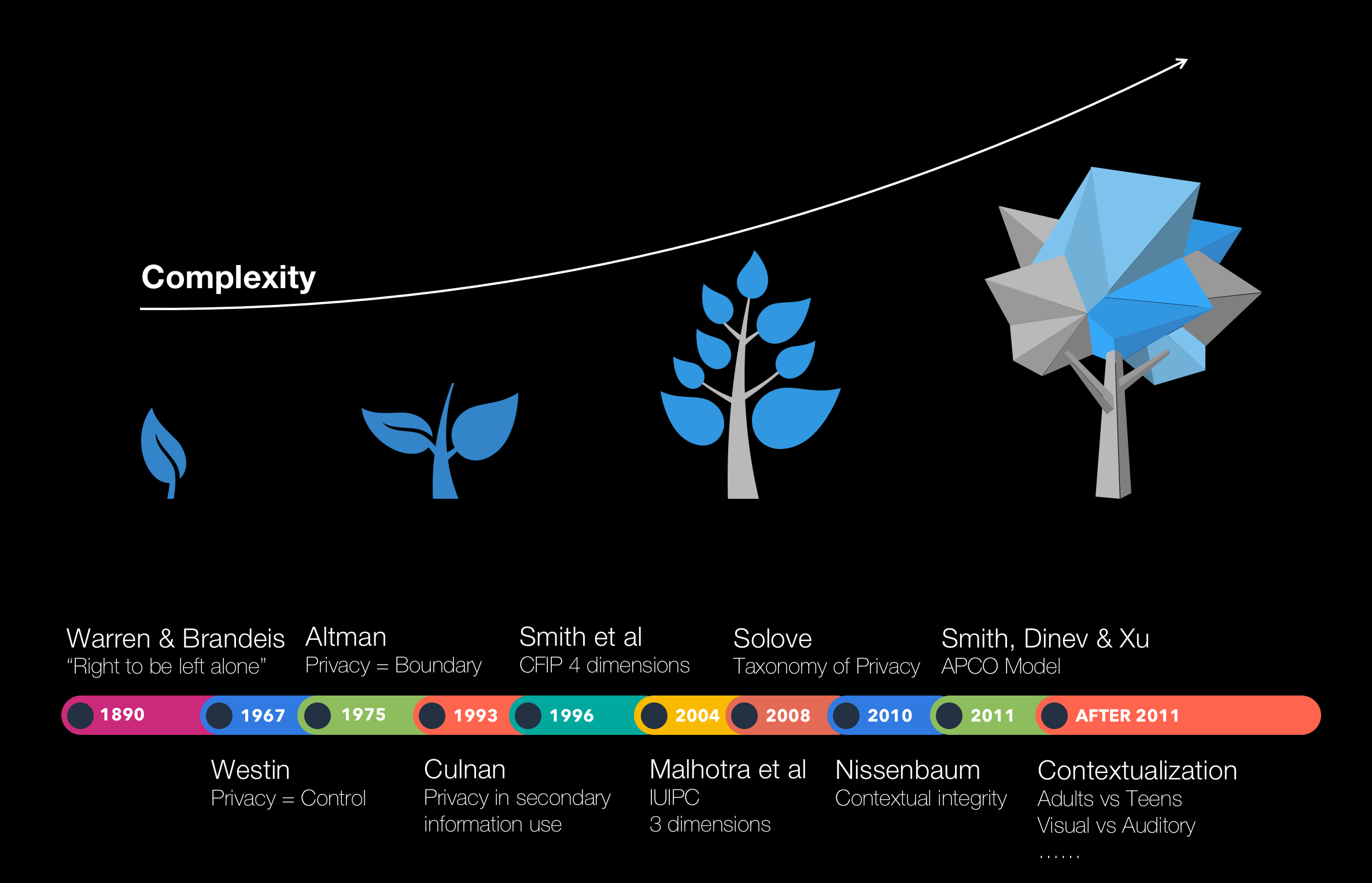
Heng Xu, co-director of the Robust Analytics Lab, will give a talk at the iSchool, University of Maryland, College Park, titled “Privacy Contextualization: the Good, the Bad, and the Ugly”. Visit the event webpage for more information about the talk.
Time: 11am – 12pm, Tuesday, September 25, 2018
Location: 2116 Hornbake Bldg, South Wing, University of Maryland, College Park
Abstract
As our information ecology evolves to be more digital and ubiquitous, we are moving from a world where data collection and processing is siloed and specialized, to a world where everyday individuals produce massive trails of data and consume considerable amounts of data products. When this complex ecosystem is forming around individuals, organizations, and data, it is important to understand how the human-technology frontier is shaping up in data practices to balance between technological design and the human/societal needs. A major aspect of this human-technology frontier is the concerns on information privacy, which is becoming an increasingly critical and global challenge for many stakeholders including business leaders, IT professionals, privacy activists, and government regulators. In this talk, I will first describe how extensive research efforts were spent on refining the highly contextualized nature of privacy, and revealing factors that affect people’s privacy concerns, from cognition to emotion to environment. Then I will argue that, while we can continue down this line to keep adding factors towards an overcomplicated model of privacy: the more researchers try to “refine” a construct to precision, the easier it is for them to focus too much on “siloed” factors and lose sight of the “big picture”, eventually departing from what real-world people think the construct means. What I propose in my ongoing work is to develop practical models that are not designed to serve as a comprehensive explanation for the construct of privacy, but to capture most concerns by most individuals in most situations, towards the goal of actionable design guidelines for more usable privacy solutions in practice.