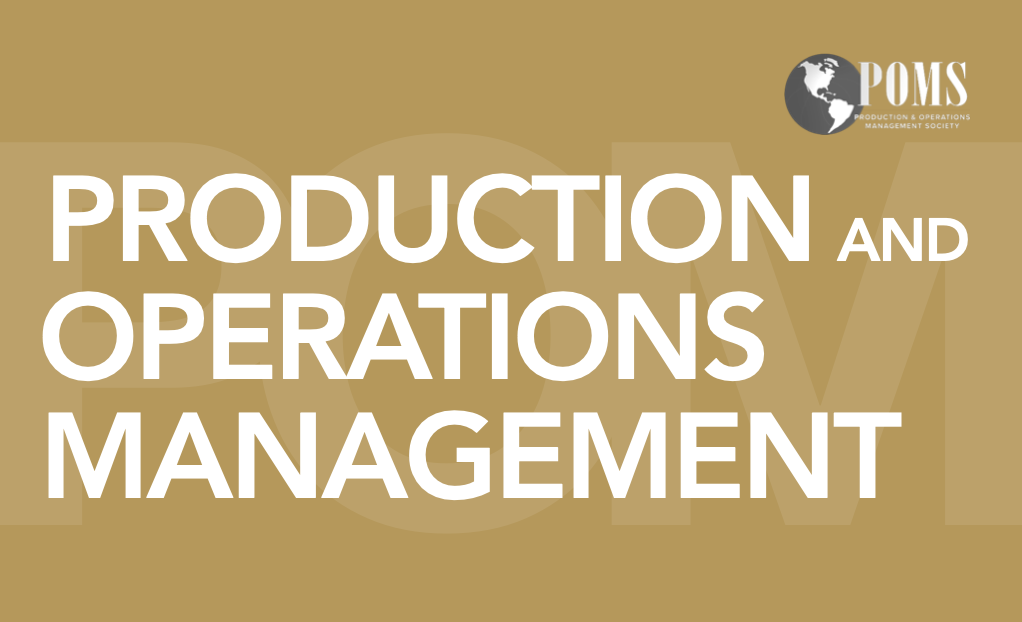
Goal Orientation for Fair Machine Learning Algorithms
In an article forthcoming at Production and Operations Management, we discuss the importance of goal orientation for fairness-aware machine learning algorithms.
In an article forthcoming at Production and Operations Management, we discuss the importance of goal orientation for fairness-aware machine learning algorithms.
In an article forthcoming at Information Systems Research, we discuss a new onto-epistemological perspective for understanding the privacy phenomena.
In an article at Personnel Psychology, we study the potential of machine learning for reducing adverse impact in personnel selection.
In an article forthcoming at Information Systems Research, we drew from the recent advances in machine learning to study the fairness of ratemaking methods for catastrophe insurance.
By recounting the trajectory of privacy scholarship and the perplexing research questions that have arisen, we discuss ways to make our extant theories better aligned with the changing landscape of privacy regulation and industry practice.
In an article published at Management Science, we developed a framework for assessing how context-oriented nuances influence privacy concerns.
In an article published at Management Science, we examined the potentially disparate impact of privacy protection on underprivileged sub-populations.
In an article published at MIS Quarterly, we studied the feasibility of leveraging advanced analytical techniques to collectively reason about the conflicting findings in behavioral research.
In an article published at Psychological Methods, we leveraged the recent advances in theoretical machine learning to develop a novel method for disentangling heterogeneity in meta-analysis.
In an article published at Journal of the Association for Information Systems, we studied the circumstances that lead to employees committing malicious acts against organizational digital assets.
In an article published at Journal of Management, we reviewed the common issues that threaten the validity of inferences drawn in empirical studies from analyzing organic data.
In an article published at Medical Care, we demonstrated that the integration of demographics attributes such as race in public health datasets could lead to privacy disparity if not carefully treated.