Testing of Reverse Causality Using Semi-Supervised Learning
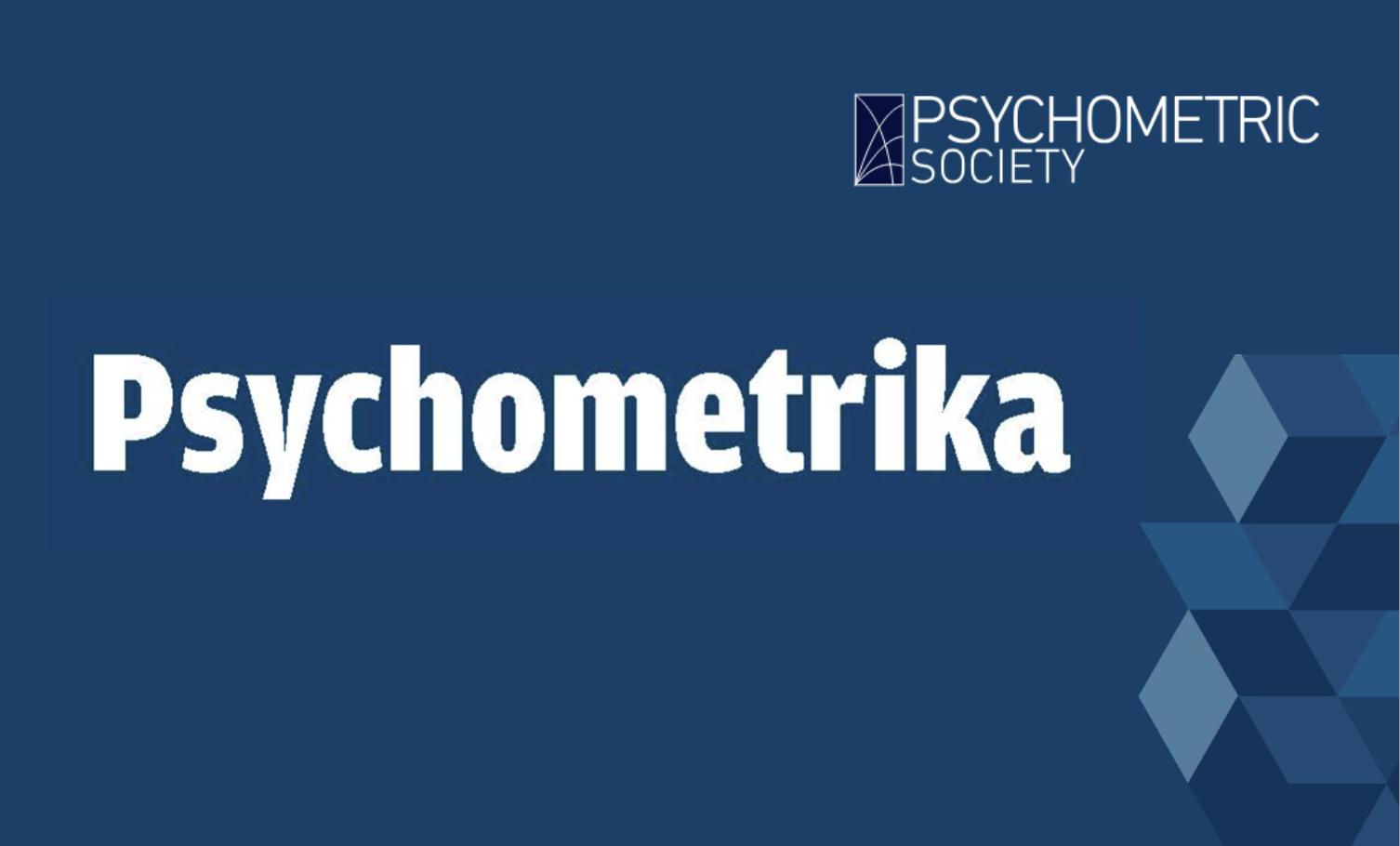
Two potential obstacles stand between the observation of a statistical correlation and the design (and deployment) of an effective intervention, omitted variable bias and reverse causality. Whereas the former has received ample attention, comparably scant focus has been devoted to the latter in the methodological literature. Many existing methods for reverse causality testing commence by postulating a structural model that may suffer from widely recognized issues such as the difficulty of properly setting temporal lags, which are critical to model validity. In this paper, we draw upon advances in machine learning, specifically the recently established link between causal direction and the effectiveness of semi-supervised learning algorithms, to develop a novel method for reverse causality testing that circumvents many of the assumptions required by traditional methods.
The paper, “Testing of Reverse Causality Using Semi-Supervised Machine Learning”, co-authored by Nan Zhang, Heng Xu, Manuel Vaulon, and Zhen Zhang, was accepted for publication at Psychometrika.
We will post a link to the article once it is published online.